Sales & Data Analytics
Sales and data analytics play a crucial role in helping businesses make informed decisions, optimize their sales strategies, and enhance overall performance. Here are key aspects of how sales and data analytics intersect
Customer Segmentation:
- Use data analytics to identify and segment customers based on various criteria, such as demographics, buying behavior, and preferences. This helps tailor sales strategies to specific customer segments.
Predictive Analytics:
- Predictive analytics can forecast future sales trends and customer behavior. By analyzing historical data, businesses can identify patterns and make proactive decisions to capitalize on opportunities or mitigate risks.
Sales Forecasting:
- Leverage data analytics to create accurate sales forecasts. This involves analyzing past sales data, market trends, and external factors to predict future sales performance.
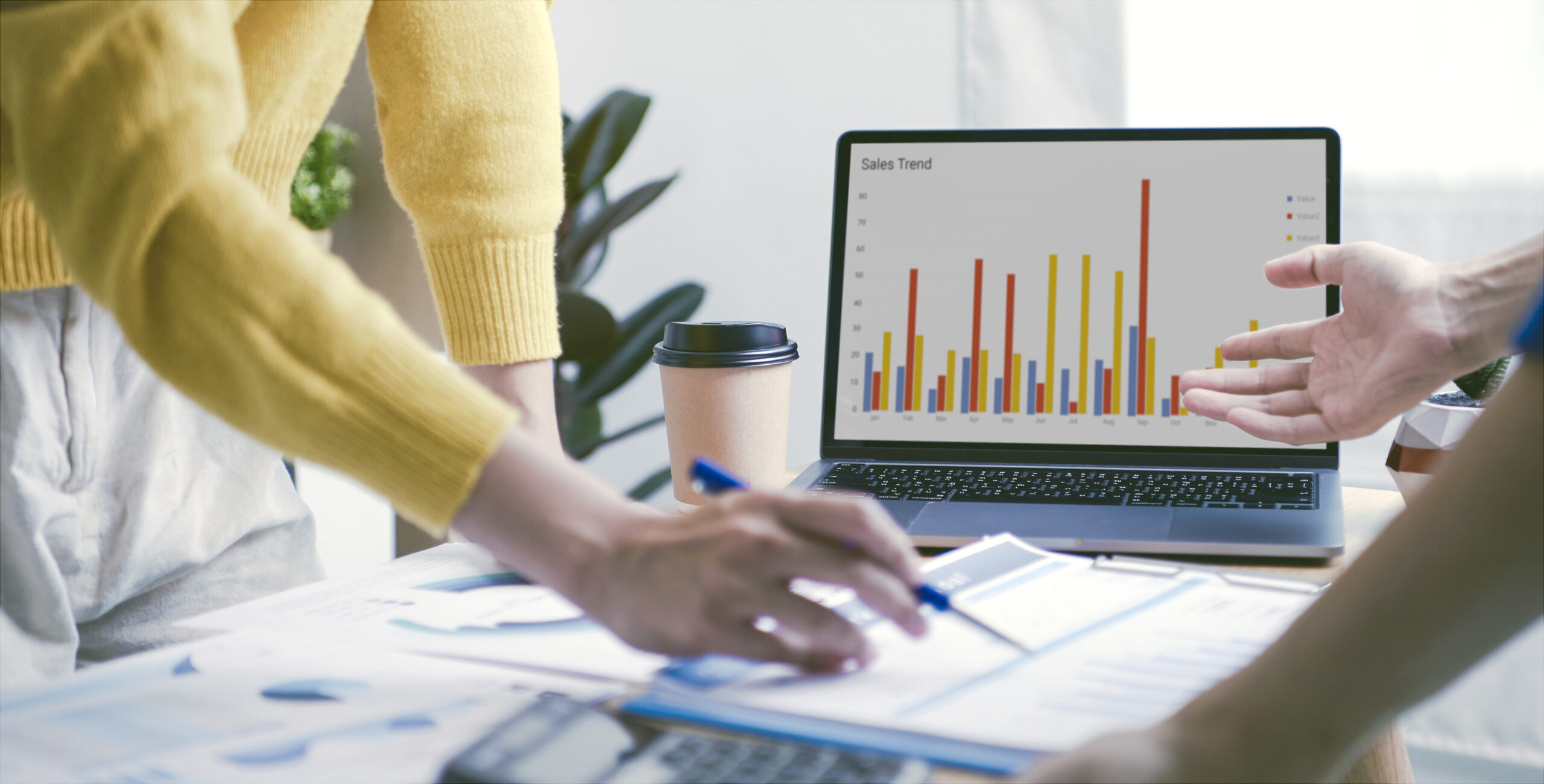
Customer Lifetime Value (CLV) Analysis
Determine the CLV by analyzing customer data over time. This information helps in optimizing customer acquisition costs and tailoring strategies to maximize long-term value.
Predictive Analytics
- Predictive analytics can forecast future sales trends and customer behavior. By analyzing historical data, businesses can identify patterns and make proactive decisions to capitalize on opportunities or mitigate risks.
-